Recap
Using Machine Learning to Chart Worker Behavior
NYU SPS DAUS Student Leverages Machine Learning to Forecast Employee Turnover at Tech Firms
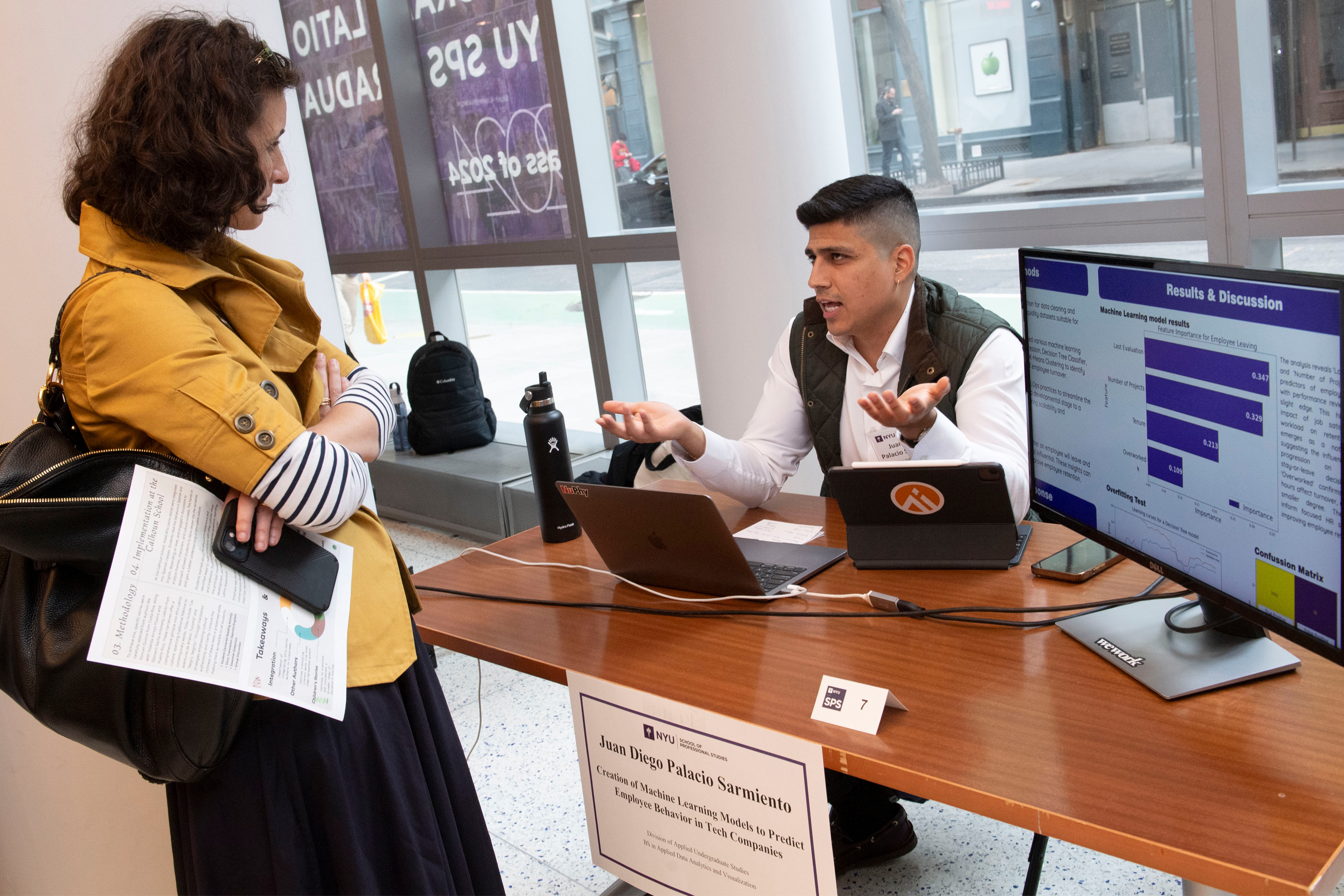
Juan has the unique ability of allowing data to shape his research while at the same time interpreting broader implications. It was impressive seeing these critical research skills together.”
Joseph Panzarella
Adjunct Assistant Professor
NYU SPS Division of Programs in Business
Using Machine Learning to Chart Worker Behavior
<p>For companies of all sizes, retaining valued and talented employees can be a challenge that can have a significant impact on the growth and future existion of a business. For his NYU SPS Capstone Fair project, Juan Diego Palacio Sarmiento leveraged his degree skills in applied data and analytics, and advanced machine learning techniques to analyze employee turnover within technology companies.</p>
<p>The goal of the project was to help determine the key factors influencing employee decisions to leave a company. Thus, giving human resources and human capital management departments and professionals relevant data and insights to develop targeted interventions to increase employee retention rates.</p>
<p> </p>
<p style="text-align: left;"><span class="p-body">Using skills learned studying for his <a href="/content/sps-nyu/explore/degrees-and-programs/bs-in-applied-data-analytics-and-visualization.html" target="_blank">BS in Data Analytics and Visualization (STEM)</a>, Sarmiento conducted a comprehensive analysis using decision tree classifiers, logistic regression, multinomial Naive Bayes, and K-means clustering on a curated dataset to discern key factors influencing employees’ decisions to leave tech companies. Sarmiento's decision tree classifier emerged superior, with an accuracy of 94% and a precision of 89%, underscoring the importance of performance evaluations and workload balance in retention strategies.</span></p>
<p style="text-align: left;"><span class="p-body">Sarmiento's use of confusion matrix analysis revealed a strong model specificity with minimal false negatives and positives, enhancing the practical utility of machine learning for HR departments. His project's integration with machine learning operations practices ensured a seamless transition to a production environment, bolstering both scalability and maintainability. He intends to incorporate series analysis and granular demographic data to refine predictive accuracy even further in future projects. </span></p>
<p> </p>
Learn More About the NYU SPS Capstone Program
<p><span class="p-body"><a href="/content/sps-nyu/experience/how-youll-learn/consulting-practicums.html">NYU School of Professional Studies’ Capstone Program</a> provides graduate and undergraduate students the opportunity to apply their classroom learning in complex, real-world environments. The final product exemplifies the volume and depth of theoretical knowledge gained in the classroom. Held each spring, the annual Capstone Fair features projects that embody the School's innovative spirit. We invite students graduating in the spring, fall, and summer graduates to participate. Students apply via form, and faculty can nominate individuals or teams.</span></p>